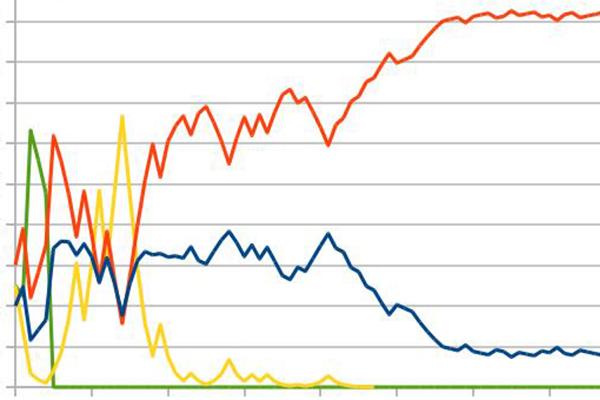
Causality lies at the heart of many scientific research endeavors, including Statistics, Biostatistics, Epidemiology, Economics, Computer Science, Data Science, Sociology, Political Science, etc. In recent years, the use of Bayesian methods in causal inference has drawn more attention in both randomized trials and observational studies. Bayesian ideas have spread over in many topics of causal inference, from the adaptive trial design, to missing data framework and graphical models. This Bayesian Causal Inference Workshop intends to bring together interdisciplinary thought leaders and researchers who are interested in developing and applying Bayesian methodology to infer causal relationship from experimental and non-experimental data. We also encourage junior researchers to participate the discussion and develop their professional network.