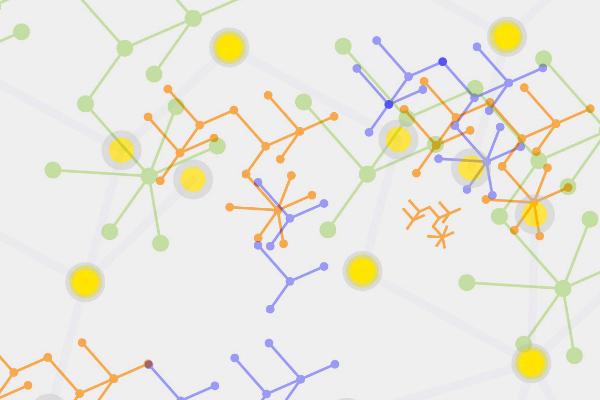
Additional conference materials can be found at: https://www.asc.ohio-state.edu/kurtek.1/cbms.html
Topic Area
This Conference Board of the Mathematical Sciences (CBMS) conference will feature an intensive lecture series on elastic methods for statistical analysis of functional and shape data, using tools from Riemannian geometry, Hilbert space methods, and computational science. The main focus of this conference is on geometric approaches, especially on using elastic Riemannian metrics with desired invariance properties, and square-root representations that simplify computations. These approaches allow joint registration and statistical analysis of functional data, and are termed elastic for that reason. The statistical goals include comparisons, summarization, clustering, modeling, and testing of functional and shape data objects.
Primary Lecturer
Prof. Anuj Srivastava is a Professor of Statistics and a Distinguished Research Professor at Florida State University (FSU) in Tallahassee, FL. His main expertise lies in the use of techniques from algebra and differential geometry in deriving statistical inferences on nonlinear manifolds. Specifically, along with his colleagues, he has developed comprehensive Riemannian frameworks for shape analysis of objects, including scalar functions, Euclidean curves, 2D surfaces, and neuronal trees. He is an author, along with Prof. Eric Klassen of FSU, of a recently published Springer textbook on Functional and Shape Data Analysis. He has also published more than 200 papers in refereed journals and proceedings of refereed international conferences. He is a fellow of the IEEE, IAPR, and ASA.
Additional Lecturers
Prof. Eric Klassen, Department of Mathematics, Florida State University
Prof. Veera Baladandayuthapani, Department of Biostatistics, University of Texas MD Anderson Cancer Center
Prof. Laurent Younes, Department of Applied Mathematics and Statistics, Johns Hopkins University
Prof. Zhengwu Zhang, Department of Biostatistics and Computational Biology, University of Rochester
We gratefully acknowledge funding and support from the National Science Foundation CBMS grant, the Mathematics Research Institute, the Mathematical Biosciences Institute, the Department of Statistics at Ohio State, and the NSF TRIPODS grant.
Organizers
Sebastian Kurtek
Department of Statistics
The Ohio State University
Facundo Memoli
Department of Mathematics
The Ohio State University
Yusu Wang
Computer Science and Engineering
The Ohio State University
Tingting Zhang
Statistics
University of Virginia
Hongtu Zhu
Biostatistics
University of Texas MD Anderson Cancer Center
Talks
Bayesian Models for Richly Structured Data in Biomedicine
Veera Baladandayuthapani (Department of Biostatistics, UT MD Anderson Cancer Center)
Modern scientific endeavors generate high-throughput, multi-model datasets of different sizes, formats, and structures at a single subject-level. In the context of biomedicine, such data include multi-platform genomics, proteomics and imaging; and each of these distinct data types provides a different, partly independent and complementary, high-resolution view of various biological processes. Modeling and inference in such studies is challenging, not only due to high dimensionality, but also due to presence of rich structured dependencies such as serial, spatial, graphical, functional and shape-based correlations. This talk will cover probabilistic frameworks that acknowledge and exploit these inherent complex structural relationships to develop regression and clustering models, that extract maximal information from such data. These approaches will be illustrated using several biomedical case examples especially in oncology.
Statistical Models
Karthik Bharath (Statistics, Florida State University)
- Statistical modeling of functional data: Karcher mean, multiple registration, fPCA in quotient space.
- Curves on manifolds: smoothing splines.
- Trajectories on Manifolds: issues; transported SRVFs, vector bundle representations, tangent bundle representation.
Generalizations of the Square Root Velocity Framework to Trajectories in Manifolds
Eric Klassen (Mathematics, Florida State University)
In previous talks, SRVF method has been discussed as a powerful and efficient way to analyze collections of curves in a Euclidean space. However, in many applications the data to be analyzed consists of trajectories in a manifold, rather than in a Euclidean space. Examples include hurricane paths on the surface of the earth, paths of covariance matrices that arise in the study of brain connectivity, and paths of images that lie in a shape space. The comparison of curves in a manifold is more subtle than in a Euclidean space, because one cannot subtract velocity vectors that lie in two different tangent spaces! In this talk, we discuss several methods of adapting the SRVF method to this situation, and comment on the advantages and disadvantages of each method.
Fundamental Mathematical Formulations, Recent Progress and Open Problems
Eric Klassen (Statistics, Florida State University)
- scalar FDA
- characterization of orbits
- existence of optimal matching for piecewise-linear curves
- C1 functions
Generalizations of the Square Root Velocity Framework to Trajectories in Manifolds
Anuj Srivastava (Mathematics, Florida State University)
In previous talks, SRVF method has been discussed as a powerful and efficient way to analyze collections of curves in a Euclidean space. However, in many applications the data to be analyzed consists of trajectories in a manifold, rather than in a Euclidean space. Examples include hurricane paths on the surface of the earth, paths of covariance matrices that arise in the study of brain connectivity, and paths of images that lie in a shape space. The comparison of curves in a manifold is more subtle than in a Euclidean space, because one cannot subtract velocity vectors that lie in two different tangent spaces! In this talk, we discuss several methods of adapting the SRVF method to this situation, and comment on the advantages and disadvantages of each method.
Introduction and Background
Anuj Srivastava (Statistics, Florida State University)
- Introduction and motivation.
- Function Spaces: norms, Hilbert space; L2 metric; complete orthonormal basis; sample mean, sample covariance; functional Principal Component Analysis (fPCA); functional regression models; generative models for functional data; least squares curve fitting.
Elastic Shape Analysis of Curves
Anuj Srivastava (Statistics, Florida State University)
- Goals and motivation; Past approaches in shape analysis; shape-preserving group actions; Kendall's shape analysis; active shape models; registration problem; non-elastic framework.
- Elastic Riemannian metric; SRVF; open curves and closed curves; shape geodesics.
- Statistics of shapes: sample mean, shape PCA, shape models, clustering and classification; symmetric shapes.
Fundamental Mathematical Formulations, Recent Progress and Open Problems
Anuj Srivastava (Statistics, Florida State University)
- scalar FDA
- characterization of orbits
- existence of optimal matching for piecewise-linear curves
- C1 functions
Elastic Shape Analysis of Surfaces
Anuj Srivastava (Statistics, Florida State University)
- Goals and motivation; past approaches; Iterative Closest Point (ICP) algorithm.
- Representations of surfaces: coordinate functions, gradient field, surface normal, 1nd fundamental form, 2nd fundamental form.
- Registration problem; elastic Riemannian metric; square-root normal fields (SRNFs); inversion problem; shape geodesics.
- Statistics of shapes: sample mean, shape PCA, shape models, clustering and classification; symmetric shapes.
Elastic Functional Data Analysis
Anuj Srivastava (Statistics, Florida State University)
- Background from geometry and algebra: manifolds, Riemannian metric, geodesics, exponential and inverse exponential map, Karcher/Frechet means; functional spaces of interest: set of probability density functions (pdfs), set of warping functions, group, group actions, quotient spaces.
- Phase variability and the registration problem; L2 metric and pinching problem; dynamic programming algorithm; penalized L2 metric.
- Invariance, square-root velocity function (SRVF); Fisher-Rao Riemannian metric; change of variables; registration using SRVFs; exact solution and approximation; quotient space metric, amplitude and phase distances.
Statistical Models
Anuj Srivastava (Statistics, Florida State University)
- Statistical modeling of functional data: Karcher mean, multiple registration, fPCA in quotient space.
- Curves on manifolds: smoothing splines.
- Trajectories on Manifolds: issues; transported SRVFs, vector bundle representations, tangent bundle representation.
Introduction to Metric Registration
Laurent Younes (Applied Mathematics and Statistics, Johns Hopkins University)
Registration (for curves, surfaces or images) can be seen as a representation of a shape dataset as a subset of the diffeomorphism group, each shape in the dataset being associated with an optimal deformation of a template. We will in particular focus on methods that compute this deformation by optimizing a distance over diffeomorphisms under the constraint of mapping a template to a target, and such that the minimized distance is in turn a metric in shape space. These “metric registration� methods include a large spectrum of algorithms deriving from the “large deformation diffeomorphic metric mapping� (LDDMM) framework, and “metamorphosis� in which non-diffeomorphic shape variations are also allowed. We will describe mathematical foundations and algorithms, and the various forms that these methods may take in relation to applications.
Geometry-based Brain Structural Connectome Analysis
Zhengwu Zhang (Department of Biostatistics and Computational Biology, University of Rochester)
Advanced brain imaging techniques make it possible to measure individuals' structural connectomes in large cohort studies non-invasively. The structural connectome is initially shaped by genetics and subsequently refined by the environment. It is extremely interesting to study structural connectomes and their relationships to environmental factors or human traits, which motivates the routine collection of high-resolution connectomes in large human studies (e.g., the Human Connectome Project and UK Biobank). However, there is a fundamental gap between the state of the art in image acquisition and the tools available to reconstruct and analyze the connectome data, due to the complexity of data. This lecture aims at introducing our recent effort in the thread. More specifically, we will introduce a population-based structural connectome (PSC) mapping framework to reproducibly extract binary networks, weighted networks, and streamline-based brain connectomes. In addition, various novel methods of analyzing the outputs of PSC will be introduced. We will also introduce a user-friendly software package that implements the PSC and the analysis methods.