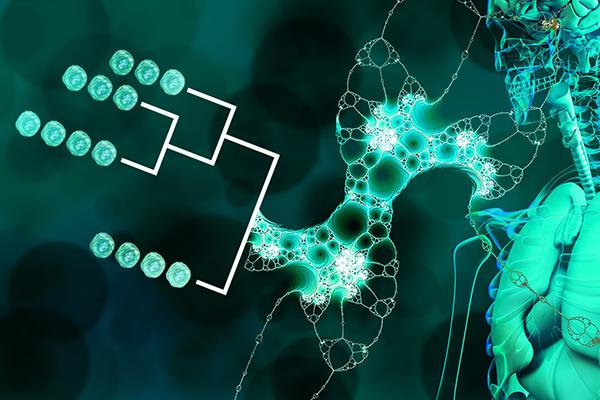
Cancer is a heterogeneous disease, with variation across individuals with regard to aspects such as rate of progression, response to treatment, and survival time. Although the polyclonal nature of many cancers and the role of evolutionary processes in tumor progression was first established in the late 1970’s, increased attention has recently been given to these processes as their potential role in clinical outcomes has become more widely recognized. This has in part been motivated by the availability of data at a finer scale than ever before, though technological developments such as single-cell sequencing. Given the availability of such data, there is now a need for models and methods that can produce realistic pictures of the evolutionary history of a tumor. In this workshop, we explore the use of mathematical and statistical models that include an evolutionary component to study cancer at various scales, including evolution that occurs within individual tumors, across transmissible cancers, and within populations, possibly in conjunction with environmental and genetic factors.