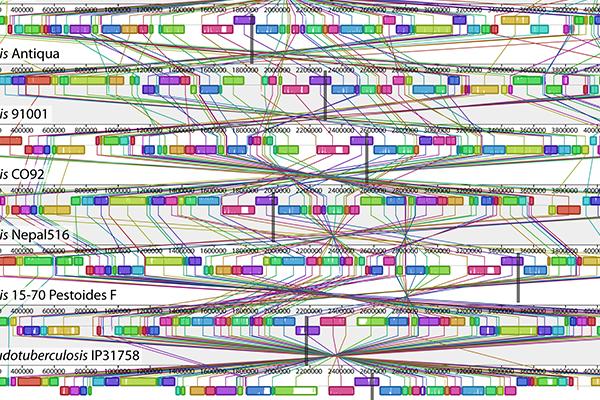
The field of Genetic Epidemiology has historically focused on the inheritance of genetic factors and phenotypes within families. However, the increase in ever improving technologies brought a shift from familial study designs to genome wide association studies (GWAS) utilizing samples of unrelated individuals. While GWAS has yielded greater knowledge of genomic structure and disease associated variants, the estimated effect sizes are small and often to not explain a large proportion of disease heritability. One of the explanations for the missing heritability is that the variants identified in GWAS are common (> 5%) and thus we are missing an entire class of variation (rare) that substantially contributes to disease risk. The innovation of next-generation sequencing technology made the comprehensive discovery of rare variants feasible, however the sample size of unrelated individuals needed to identify associations between these rare variants and diseases is in the thousands (> 10,000 samples are necessary to detect a variant showing evidence of modest association with minor allele frequency 0.1%). While sequencing costs have decreased, the financial burden is still nontrivial and sample heterogeneity can easily confound results. Thus, efficient study designs and improved statistical approaches are necessary to untangle the contribution of rare variation to complex disease. Family studies have always been robust to confounding and a powerful approach for identifying genetic variation. In the age of sequencing, family studies are again an appealing approach for studying the relationship between complex disease and genetic variation.
This workshop will focus on the use of family studies in the hunt for disease associated genes, include the development of novel methodologies and statistics for assessing variant disease relationships as well as the important role of the family study design in a clinical sequencing setting.