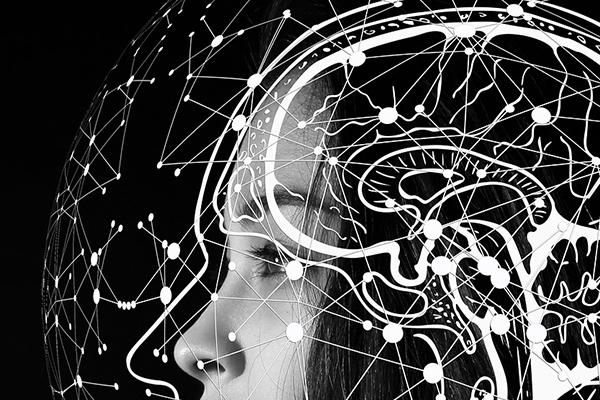
Mathematical and computational methods are critical to conduct research in many areas of biology, such as genomics, molecular biology, cell biology, developmental biology, neuroscience, ecology and evolution. Conversely, biology is providing new challenges that drive the development of novel mathematical and computational methods. This workshop brings together world experts to present and discuss recent development of mathematical methods that arise in biological sciences. The topics include deterministic, stochastic, hybrid, multiscale modeling methods, as well as analytical and computational methods.
This event will be held virtually using Zoom. Please register below.