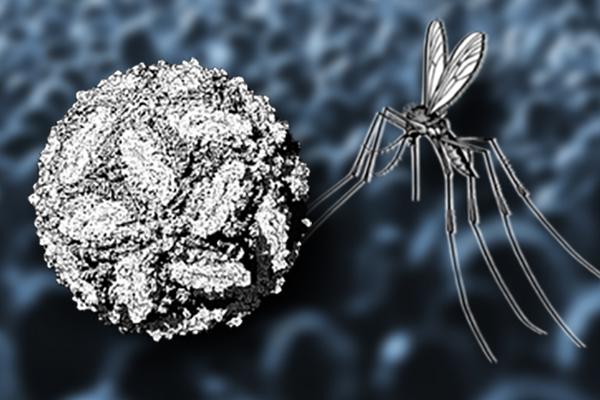
Modern methods of genomic data collection reveal a great heterogeneity and diversity among strains of pathogens with different genotypes infecting hosts with significant differences in virulence, immunogenicity, and antigenic variation on a micro-scale (Workshop 1). The presence/persistence of specific different sub-groups of such pathogens depends heavily on macro-scale interactions of various human and animal hosts, travel patterns, environment, and intervention strategies (Workshop 2-3). In order to properly understand the main drivers of transmission of infectious disease, these ecological, molecular, and immunological factors need to be analyzed together, and their joint correct characterization requires a comprehensive interdisciplinary and multi-scale modeling approach. We expect that different infection outcomes are the result of the interplay of events at organ tissue cellular and molecular as well as ecosystem scales over the time course of minutes to years. A recent example is the Zika virus epidemic where a 3-5 days infection of a pregnant woman can result in birth defects lasting for the newborn’s lifetime. In order to study such problems, the unifying modeling framework for simultaneously analyzing multiple scales of empirical information from the level of single cells to organs, organisms, population and ecosystems appears necessary.
However, integrating the information from the micro- and macro-scales presents a great scientific challenge as the data on different scales is generated by different mechanisms and comes with different characteristics and uncertainties. In fact, integration across scales can be seen as the fundamental challenge for biology in the 21st century. The mathematical challenges raised are truly substantial, as the integration requires dealing with multiple temporal and spatial scales, as well as organizational scales. If scales in time and space are well separated, there exist, at least for deterministic systems, methods based on perturbation approaches that can be used. However, even these approaches are difficult to apply to systems with the kinds of underlying heterogeneities that will be found in systems with diseases. There are methods for averaging over heterogeneities but these methods as well have limitations. And, the different time scales of observations and the different framework that models at different scales use present substantial challenges.
This workshop will bring together scientists from multiple disciplines, to exchange ideas about new perspectives for the quantification of within-host dynamics and between-host transmission of infectious disease as well as integration over multiple temporal and spatial scales. Approaches that consider how to integrate approaches at different scales that range from deterministic to stochastic to computational will be a central theme, including how to incorporate data and how to deal with situations with limited data. It is assumed that a representative subset of participants in Workshops 1-3 will also participate. In the context of some of the problems Participants will discuss novel molecular and ecological data that has become available ('omic, clinical, entomological, and epidemiological data), and will discuss modeling perspectives that will allow their integration beyond traditional epidemiological models of transmission with the goals of improving public health practice and policies.