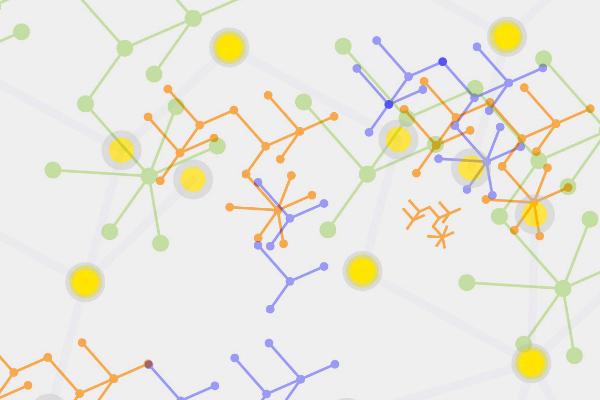
The last few years have seen the rapid development of mathematical methods for the analysis of shape data arising in biology and computer vision applications. Recently developed tools coming from the fields of optimal transport and topological data analysis have proved to be particularly successful for these tasks. The goal of this conference is to bring together researchers from these communities to share ideas and to foster collaboration between them. Talks will focus on computational and theoretical aspects as well as on applications, with a focus on shape analysis.
View the TGDA@OSU conference website here: https://tgda.osu.edu/ot-tda-workshop/
Recordings of the talks are located in the Abstracts Tab below.
Organizers
Facundo Memoli
Department of Mathematics
The Ohio State University
memoli@math.osu.edu
Thomas Needham
Department of Mathematics
Florida State University
tneedham@fsu.edu
Jose Perea
Department of Computational Mathematics, Science and Engineering and Department of Mathematics
Michigan State University
joperea@msu.edu
Nicolas Garcia Trillos
Department of Statistics
University of Wisconsin
nicolasgarcia@stat.wisc.edu
Schedule
Time | Session |
---|---|
08:45 AM 09:00 AM |
Opening Remarks |
Morning Session |
Moderated by Jose Perea |
09:00 AM 09:40 AM |
Robert McCann - When Do Interacting Organisms Gravitate to the Vertices of a Regular Simplex? |
09:40 AM 09:50 AM |
Break |
09:50 AM 10:30 AM |
Lori Ziegelmeier - Using Topology to Measure Dynamics of Time-Varying Systems |
10:30 AM 10:40 AM |
Break |
10:40 AM 11:20 AM |
Sayan Mukherjee - Machine Learning for 3D Imaging |
11:20 PM 01:00 PM |
Lunch Break |
Afternoon Session |
Moderated by Jose Perea |
01:00 PM 01:40 PM |
Henry Adams - Metric reconstruction via optimal transport |
01:40 PM 01:50 PM |
Break |
01:50 PM 02:30 PM |
Theo Lacombe - Spaces of persistence diagrams and measures, an optimal transport viewpoint |
Time | Session |
---|---|
Morning Session |
Moderated by Facundo Memoli |
09:00 AM 09:40 AM |
Justin Solomon - Sampling-Based Algorithms for Optimal Transport Problems |
09:40 AM 09:50 AM |
Break |
09:50 AM 10:30 AM |
Guido Montufar - Optimal Transport to a Variety |
10:30 AM 10:40 AM |
Break |
10:40 AM 11:20 AM |
Katy Craig - Gradient Flows in the Wasserstein Metric: From Discrete to Continuum via Regularization |
11:20 PM 01:00 PM |
Lunch Break |
Afternoon Session |
Moderated by Nicolas Garcia Trillos |
01:00 PM 01:40 PM |
Brittany Terese Fasy - Finite Representations of Shapes in Euclidean Space |
01:40 PM 01:50 PM |
Break |
01:50 PM 02:30 PM |
Justin Curry - Counting Problems in Persistence |
Time | Session |
---|---|
Morning Session |
Moderated by Thomas Needham |
09:00 AM 09:40 AM |
Chao Chen - Topological Loss for Deep Generative Models |
09:40 AM 09:50 AM |
Break |
09:50 AM 10:30 AM |
Radmila Sazdanovic - Applied topology methods in knot theory |
10:30 AM 10:40 AM |
Break |
10:40 AM 11:20 AM |
Marcel Klatt - Empirical (Regularized) Optimal Transport: Statistical Theory and Applications |
11:20 PM 01:00 PM |
Lunch Break |
Afternoon Session |
Moderated by Thomas Needham |
01:00 PM 01:40 PM |
Christoph Weitkamp - Gromov-Wasserstein Distance based Object Matching: Asymptotic Inference |
01:40 PM 01:50 PM |
Break |
01:50 PM 02:30 PM |
Claire Brecheteau - Robust approximation of compact sets with unions of ellipsoids. Application to clustering |
Time | Session |
---|---|
Morning Session |
Moderated by Facundo Memoli |
09:00 AM 09:40 AM |
Julie Delon - A Wasserstein-type distance in the space of Gaussian Mixture Models |
09:40 AM 09:50 AM |
Break |
09:50 AM 10:30 AM |
Sinho Chewi - Gradient descent algorithms for Bures-Wasserstein barycenters |
10:30 AM 10:40 AM |
Break |
10:40 AM 11:20 AM |
Edward Chien - Spectral proxies for Ollivier-Ricci curvature on graphs |
11:20 PM 01:00 PM |
Lunch Break |
Afternoon Session |
Moderated by Thomas Needham |
01:00 PM 01:40 PM |
Samir Chowdhury - A Riemannian framework for Gromov-Wasserstein averaging with applications to neuroimaging |
01:40 PM 01:50 PM |
Break |
01:50 PM 02:30 PM |
Hongteng Xu - Gromov-Wasserstein Factorization for Graph Representation |
Time | Session |
---|---|
Morning Session |
Moderated by Nicolas Garcia Trillos |
09:00 AM 09:40 AM |
Pavan Turaga - Topological methods for robust human activity modeling |
09:40 AM 09:50 AM |
Break |
09:50 AM 10:30 AM |
Jessi Cisewski-Kehe - Analyzing Data Full of Holes with Topological Data Analysis |
10:30 AM 10:40 AM |
Break |
10:40 AM 11:20 AM |
Bei Wang - Topology as a knob for machine learning |
11:20 PM 01:00 PM |
Lunch Break |
Afternoon Session |
Moderated by Nicolas Garcia Trillos |
01:00 PM 01:40 PM |
Xianfeng David Gu - An Optimal Transport View of Generative Models in Deep Learning |
01:40 PM 01:50 PM |
Break |
01:50 PM 02:30 PM |
Varun Jog - A medley of geometry, optimal transport, and machine learning |
02:30 PM 03:00 PM |
Closing Remarks |
Speakers
Name | Affiliation | |
---|---|---|
Henry Adams | Colorado State University | henry.adams@colostate.edu |
Claire Brecheteau | Ecole Centrale Nantes | claire.brecheteau@ec-nantes.fr |
Chao Chen | Stony Brook University | chao.chen.cchen@gmail.com |
Sinho Chewi | MIT | schewi@mit.edu |
Edward Chien | MIT | edchien@mit.edu |
Samir Chowdhury | Stanford University | samirc@stanford.edu |
Jessi Cisewski-Kehe | Yale University | jessica.cisewski@yale.edu |
Brittany Terese Fasy | Montana State University | brittany@cs.montana.edu |
Katy Craig | University of California, Santa Barbara | kcraig@math.ucsb.edu |
Justin Curry | University at Albany SUNY | jmcurry@albany.edu |
Julie Delon | Universite Paris Descartes | prenom.nom@parisdescartes.fr |
Xianfeng David Gu | Stony Brook University | gu@cs.stonybrook.edu |
Varun Jog | University of Wisconsin | vjog@wisc.edu |
Marcel Klatt | University of Göttingen | mklatt@mathematik.uni-goettingen.de |
Theo Lacombe | Institut Polytechnique de Paris | theo.lacombe@inria.fr |
Robert McCann | University of Toronto | mccann@math.toronto.edu |
Giudo Montufar | UCLA | montufar@math.ucla.edu |
Sayan Mukherjee | Duke University | mukhe002@duke.edu |
Radmila Sazdanovic | North Carolina State University | rsazdanovic@ncsu.edu |
Justin Solomon | MIT | jsolomon@mit.edu |
Pavan Turaga | Arizona State University | pturaga@asu.edu |
Bei Wang | University of Utah | beiwang@sci.utah.edu |
Christoph Weitkamp | Georg-August-Universitat, Gottingen | c.weitkamp@stud.uni-goettingen.de |
Hongteng Xu | Duke University & Infinia ML, Inc. | hongteng.xu@duke.edu |
Lori Ziegelmeier | Macalester College | lziegel1@macalester.edu |
Henry Adams (Colorado State University):
Metric reconstruction via optimal transport
Given a sample of points X from a metric space M, the Vietoris-Rips simplicial complex VR(X;r) at scale r>0 is a standard construction to attempt to recover M from X, up to homotopy type. A deficiency is that VR(X;r) is not metrizable if it is not locally finite, and thus does not recover metric information about M. We remedy this shortcoming by defining the Vietoris-Rips metric thickening VR^m(X;r) via the theory of optimal transport. Vertices are reinterpreted as Dirac delta masses, points in simplices are reinterpreted as convex combinations of Dirac delta masses, and distances are given by the Wasserstein distance between probability measures. When M is a Riemannian manifold, the Vietoris-Rips thickening satisfies Hausmann's theorem (VR^m(M;r) is homotopy equivalent to M for r sufficiently small) with a simpler proof: homotopy equivalence VR^m(M;r) -> M is now canonically defined as a center of mass map, and its homotopy inverse is the (now continuous) inclusion M -> VR^m(M;r). We discuss Vietoris-Rips thickenings of circles and n-spheres, and relate these constructions to Borsuk-Ulam theorems into higher-dimensional codomains. Joint work with Michal Adamaszek, John Bush, and Florian Frick.
Claire Brecheteau (Ecole Centrale Nantes):
Robust approximation of compact sets with unions of ellipsoids. Application to clustering.
I will introduce proxies for the distance function to the support of a distribution, whose sublevel sets are unions of balls or ellipsoids. I will consider rates of approximation of these proxies by their empirical counterpart, built from sample points. I will also explain how to use such estimators to cluster geometrically structured datasets.
Chao Chen (Stony Brook University):
Topological Loss for Deep Generative Models
Existing generative models (GAN or VAE) focus on generating realistic images based on CNN-derived image features, but fail to preserve the structural properties of real images. This can be fatal in applications where the underlying structure (e.g., neurons, vessels, membranes, and road networks) of the image carries crucial semantic meaning. We propose a novel GAN model that learns the topology of real images, i.e., connectedness and loopy-ness. In particular, we introduce a topological GAN loss that bridges the gap between synthetic image distribution and real image distribution in the topological feature space. By optimizing this loss, the generator produces images with the same structural topology as real images. We also propose new GAN evaluation metrics that measure the topological realism of the synthetic images. We show in experiments that our method generates synthetic images with realistic topology. We also highlight the increased performance that our method brings to downstream tasks such as segmentation.
Sinho Chewi (MIT):
Gradient descent algorithms for Bures-Wasserstein barycenters
We study first order methods to compute the barycenter of a probability distribution P over the space of probability measures with finite second moment. We develop a framework to derive global rates of convergence for both gradient descent and stochastic gradient descent despite the fact that the barycenter functional is not geodesically convex. Our analysis overcomes this technical hurdle by employing a Polyak-Lojasiewicz (PL) inequality and relies on tools from optimal transport and metric geometry. In turn, we establish a PL inequality when P is supported on the Bures-Wasserstein manifold of Gaussian probability measures. It leads to the first global rates of convergence for first order methods in this context.
Edward Chien (MIT):
Spectral proxies for Ollivier-Ricci curvature on graphs
On graphs, the Ollivier-Ricci (OR) curvature is an edge-based discretization of the classical Ricci curvature on Riemannian manifolds, formulated by replacing parallel transport with optimal transport. Recent works have shown its efficacy in analysis and clustering of social, gene expression, and economic networks, amongst others. Unfortunately, the computational burden is quite high for its calculation, making it impractical for larger networks. In this talk, I will describe our recent attempts at spectral proxies or approximations, which are orders of magnitude faster, but are still well-correlated and useful for applied scenarios. We also compare to other recently suggested proxies, such as the Forman-Ricci curvature and the Jaccard curvature.
Jessi Cisewski-Kehe (Yale University):
Analyzing Data Full of Holes with Topological Data Analysis
Data exhibiting complicated spatial structures are common in many areas of science (e.g., cosmology, biology), but can be difficult to analyze. Persistent homology is an approach within the area of Topological Data Analysis (TDA) that offers a framework to represent, visualize, and interpret complex data by extracting topological features which may be used to infer properties of the underlying structures. For example, TDA is a beneficial technique for analyzing intricate and spatially complex web-like data such as fibrin or the large-scale structure (LSS) of the Universe. The output from persistent homology, called persistence diagrams, summarizes the different order holes in the data (e.g., connected components, loops, voids). I will present a framework for inference or prediction using functional transformations of persistence diagrams and discuss how persistent homology can be used to locate cosmological voids and filament loops in the LSS of the Universe.
Samir Chowdhury (Stanford University):
A Riemannian framework for Gromov-Wasserstein averaging with applications to neuroimaging
Geometric and topological data analysis methods are increasingly being used in human neuroimaging studies to derive insights into neurobiology and behavior. We will begin by describing a novel application of optimal transport toward predicting task performance, and go on to explain why reproducing such insights across clinical populations requires statistical learning techniques such as averaging and PCA across graphs without known node correspondences. We formulate this problem using the Gromov-Wasserstein (GW) distance and present a recently-developed Riemannian framework for GW-averaging and tangent PCA. This framework permits derived network representations beyond graph geodesic distances or adjacency matrices. As an application, we show that replacing the adjacency matrix formulation in state-of-the-art implementations with a spectral representation leads to improved accuracy and runtime in graph learning tasks. Joint work with Caleb Geniesse, Facundo Mémoli, Tom Needham, and Manish Saggar.
Katy Craig (University of California, Santa Barbara):
Gradient Flows in the Wasserstein Metric: From Discrete to Continuum via Regularization
Over the past ten years, optimal transport has become a fundamental tool in statistics and machine learning: the Wasserstein metric provides a new notion of distance for classifying distributions and a rich geometry for interpolating between them. In parallel, optimal transport has led to new theoretical results on the stability and long time behavior of partial differential equations through the theory of Wasserstein gradient flows. These two lines of research recently intersected in a series of works that characterized the dynamics of training neural networks with a single hidden layer as a Wasserstein gradient flow. In this talk, I will briefly introduce the mathematical theory of Wasserstein gradient flows and describe recent results on discrete to continuum limits. In particular, I will show how passing from the discrete to continuum limit by introducing an appropriate regularization can lead to faster rates of convergence, as well as novel, deterministic particle methods for diffusive processes.
Justin Curry (University at Albany SUNY):
Counting Problems in Persistence
This talk will begin with a review of elementary constructions in topological data analysis (TDA), such as merge trees and the Elder Rule. A brief introduction to inverse problems in TDA is considered before turning to recent joint work with Catanzaro, Fasy, Lazovskis, Malen, Reiss, Wang and Zabka in https://arxiv.org/abs/1909.10623. The talk will conclude with recent combinatorial results obtained by my PhD student, Jordan DeSha, who provided a closed-form formula for the number of unbraided height equivalence classes (HECs) of embedded two-spheres with a prescribed level-set barcode. This formula establishes a conjecture outlined in the earlier paper with Catanzaro, et al.
Julie Delon (Universite Paris Descartes):
A Wasserstein-type distance in the space of Gaussian Mixture Models
In this talk we will introduce a Wasserstein-type distance on the set of Gaussian mixture models. This distance is defined by restricting the set of possible coupling measures in the optimal transport problem to Gaussian mixture models. We derive a very simple discrete formulation for this distance, which makes it suitable for high dimensional problems. We also study the corresponding multimarginal and barycenter formulations. We show some properties of this Wasserstein-type distance, and we illustrate its practical use with some examples in image processing.
Brittany Terese Fasy (Montana State University):
Finite Representations of Shapes in Euclidean Space
The persistence diagram is a topological summary that is gaining traction as a (directional) descriptor of shapes in Euclidean space. Recent work has shown that well-chosen (finite) sets of diagrams can differentiate between geometric simplicial complexes, providing a method for representing shapes using a finite set of topological descriptors. A related inverse problem is the following: given an oracle we can query for persistence diagrams, what is underlying geometric simplicial complex? This talk will explore the representation of simplicial complexes by parameterized families of persistence diagrams, along with the inverse problem of how to recover the initial simplicial complex.
Xianfeng David Gu (Stony Brook University):
An Optimal Transport View of Generative Models in Deep Learning
This work introduces an optimal transportation (OT) view of generative adversarial networks (GANs). Natural datasets have intrinsic patterns, which can be summarized as the manifold distribution principle: the distribution of a class of data is close to a low-dimensional manifold. GANs mainly accomplish two tasks: manifold learning and probability distribution transformation. The latter can be carried out using the classical OT method. From the OT perspective, the generator computes the OT map, while the discriminator computes the Wasserstein distance between the generated data distribution and the real data distribution; both can be reduced to a convex geometric optimization process. Furthermore, OT theory discovers the intrinsic collaborative—instead of competitive—relation between the generator and the discriminator, and the fundamental reason for mode collapse. We also propose a novel generative model, which uses an autoencoder (AE) for manifold learning and OT map for probability distribution transformation. This AE–OT model improves the theoretical rigor and transparency, as well as the computational stability and efficiency; in particular, it eliminates the mode collapse. The experimental results validate our hypothesis, and demonstrate the advantages of our proposed model.
Varun Jog (University of Wisconsin):
A medley of geometry, optimal transport, and machine learning
Modern machine learning algorithms are surprisingly fragile to adversarial perturbations of data. In this talk, we present some theoretical contributions towards understanding fundamental bounds on the performance of machine learning algorithms in the presence of adversaries. We shall discuss how optimal transport emerges as a natural mathematical tool to characterize "robust risk", which is a notion of risk in the adversarial machine learning literature that is analogous to Bayes risk in hypothesis testing. We shall also show how, in addition to tools from optimal transport, we may use reverse-isoperimetric inequalities from geometry to provide theoretical bounds on the sample size of estimating robust risk.
Marcel Klatt (University of Göttingen):
Empirical (Regularized) Optimal Transport: Statistical Theory and Applications
In recent years, the theory of optimal transport (OT) has found its way into data analysis. Especially regularized OT methods have encountered growing interest, as the routine use of OT in applications is still hampered by its computational complexity. Among others, the most prominent proposal is entropy regularization that serves to define an entropy regularized OT plan and a corresponding divergence also known as Sinkhorn divergence. This talk invites to a small trip through distributional limit theory for certain empirical (regularized) OT quantities defined for distributions supported on finite metric spaces. In particular, we will explore the statistical differences between asymptotic distributions for empirical non-regularized OT quantities and their regularized counterparts. Specific focus is set to the empirical regularized OT plan for which we can prove that it asymptotically follows a Gaussian law. As a consequence we discuss applications in colocalization analysis of protein interaction networks based on regularized OT. In the final part of the talk, we consider the non-regularized OT plan that is a solution to a finite dimensional basic linear program. In fact, distributional limit theory for such a quantity is not as straightforward and brings into play the combinatorial nature and the concept of degeneracy inherent in linear programming. This is joint work with Carla Tameling, Axel Munk and Yoav Zemel.
Theo Lacombe (Institut Polytechnique de Paris):
Spaces of persistence diagrams and measures, an optimal transport viewpoint
Persistence diagrams (PD) are routinely used in topological data analysis as descriptors to encode the topological properties of some object. These diagrams can be compared with a partial matching metric, sometimes called the "Wasserstein distance between persistence diagrams" due to its important similarities with the metrics used in optimal transport literature, although an explicit connection between these two formalisms was yet to come. By considering the space of persistence diagrams as a measure space, we reformulate its metrics as optimal partial transport problems and introduce a generalization of persistence diagrams, namely Radon measures supported on the upper half plane. Such measures naturally appear in topological data analysis when considering continuous representations of persistence diagrams (e.g. persistence surfaces) but also as expectations of probability distributions on the space of persistence diagrams. We will showcase the strength of this optimal-transport-based formalism on two problems arising in topological data analysis. First, we provide a characterization of convergence in the space of persistence diagrams (with respect to their standard metrics) in terms of vague convergence of measures. This result provides a powerful tool to study continuity properties in this space; in particular it gives an exhaustive characterization of continuous linear representations of persistence diagrams, a common tool used when incorporating persistence diagrams in machine learning pipelines. Second, this formalism allows us to prove new results regarding persistence diagrams in a random setting, as it enables to manipulate some limit objects such as expected persistence diagrams (that are not standard persistence diagrams) and to prove convergence rates and stability results in this context.
Robert McCann (University of Toronto):
When Do Interacting Organisms Gravitate to the Vertices of a Regular Simplex?
Flocking and swarming models which seek to explain pattern formation in mathematical biology often assume that organisms interact through a force which is attractive over large distances yet repulsive at short distances. Suppose this force is given as a difference of power laws and normalized so that its unique minimum occurs at unit separation. For a range of exponents corresponding to mild repulsion and strong attraction, we show that the minimum energy configuration is uniquely attained | apart from translations and rotations | by equidistributing the organisms over the vertices of a regular top-dimensional simplex (i.e. an equilateral triangle in two dimensions and regular tetrahedron in three).
If the attraction is not assumed to be strong, we show these configurations are at least local energy minimizers in the relevant d1 metric from optimal transportation, as are all of the other uncountably many unbalanced configurations with the same support. These therefore form stable attractors for the associated rst- and second-order dynamics. We infer the existence of phase transitions.
An ingredient from the proof with independent interest is the establishment of a simple isodiametric variance bound which characterizes regular simplices: it shows that among probability measures on Rn whose supports have at most unit diameter, the variance around the mean is maximized precisely by those measures which assign mass 1=(n + 1) to each vertex of a (unit-diameter) regular simplex. Based on preprint with Tongseok Lim at https://arxiv.org/abs/1907.13593
Guido Montufar (UCLA):
Optimal Transport to a Variety
We study the problem of minimizing the Wasserstein distance between a probability distribution and an algebraic variety. We consider the setting of finite state spaces and describe the solution depending on the choice of the ground metric and the given distribution. The Wasserstein distance between the distribution and the variety is the minimum of a linear functional over a union of transportation polytopes. We obtain a description in terms of the solutions of a finite number of systems of polynomial equations. A detailed analysis is given for the two bit independence model.
Sayan Mukherjee (Duke University):
Machine Learning for 3D Imaging
It has been a longstanding challenge in geometric morphometrics and medical imaging to infer the physical locations (or regions) of 3D shapes that are most associated with a given response variable (e.g. class labels) without needing common predefined landmarks across the shapes, computing correspondence maps between the shapes, or requiring
the shapes to be diffeomorphic to each other. In this talk, we introduce SINATRA: the first machine learning pipeline for sub-image analysis which identifies physical shape features that explain most of the variation between two classes without the aforementioned requirements. We also illustrate how the problem of 3D sub-image analysis can be mapped onto the well-studied problem of variable selection in nonlinear regression models. Here, the key insight is that tools from integral geometry and differential topology, specifically the Euler characteristic, can be used to transform a 3D mesh representation of an image or shape into a collection of vectors with minimal loss of geometric information.
Crucially, this transform is invertible. The two central statistical, computational, and mathematical innovations of our method are: (1) how to perform robust variable selection in the transformed space of vectors, and (2) how to pullback the most informative features in the transformed space to physical locations or regions on the original shapes. We highlight the utility, power, and properties of our method through detailed simulation studies, which themselves are a novel
contribution to 3D image analysis. Finally, we apply SINATRA to a dataset of mandibular molars from four different genera of primates and demonstrate the ability to identify unique morphological properties that summarize phylogeny.
Radmila Sazdanovic (North Carolina State University):
Applied topology methods in knot theory
A multitude of knot invariants, including quantum invariants and their categorifications, have been introduced to aid with characterizing and classifying knots and their topological properties. Relations between knot invariants and their relative strengths at distinguishing knots are still mostly elusive. We use Principal Component Analysis (PCA), Ball Mapper, and machine learning to examine the structure of data consisting of various polynomial knot invariants and the relations between them. Although of different origins, these methods confirm and illuminate similar substructures in knot data. These approaches also enable comparison between numerical invariants of knots such as the signature and s-invariant via their distribution within the Alexander and Jones polynomial data. This is joint work with P. Dlotko, J. Levitt, and M. Hajij.
Justin Solomon (MIT):
Sampling-Based Algorithms for Optimal Transport Problems
Sampling provides a common means of access to probability measures in Bayesian inference, streaming data processing, and other applications. In this setting, it is often impossible to access the distribution function or to make assumptions about the supports of the measures involved. In this talk, I will summarize some efforts in our research group to estimate optimal transport distances and solve derived optimization problems (e.g., barycenter estimation and barycentric regression) given only sample access to the input measures.
Pavan Turaga (Arizona State University):
Topological methods for robust human activity modeling
In this talk, we present an overview of our work in the area of activity analysis from a diverse set of modalities such as video, motion capture, wearables, and more. We show how topological descriptors derived from the time-series of human activity can lead to robust and invariant representations for a variety of problems. We also discuss our recent work in new topological featurization approaches using perturbation methods, and fusion with deep-learning. Our applications include recognition of human activities as well as uncovering underlying qualities of human activity for gait and balance-related health interventions.
Bei Wang (University of Utah):
Topology as a knob for machine learning
In this talk, we will give two examples of how topology could be used as a knob for machine learning. In the first example, topology is used as an interior knob for dimensionality reduction. In particular, via a method called the H-Isomap, topological information is used in combination with landmark Isomap to obtain homology-preserving embeddings of high-dimensional point clouds. In the second example, topology is used as an exterior knob for probing deep learning models.
Specifically, we probe a trained deep neural network by studying neuron activations (combinations of neuron firings) with a large set of input images. Using topological summaries, we study the organizational principle behind neuron activations, and how these activations are related within a layer and across layers. Using an interactive tool called TopoAct, we present visual exploration scenarios that provide valuable insights towards learned representations of an image classifier.
Christoph Weitkamp (Georg-August-Universitat, Gottingen):
Gromov-Wasserstein Distance based Object Matching: Asymptotic Inference
In this talk, we present a statistical theory for object matching based on the Gromov-Wasserstein distance. To this end, we model general objects as metric measure spaces. Based on this, we propose a simple and efficiently computable symptotic statistical test for pose invariant object discrimination. This is based on an empirical version of a ß- trimmed lower bound of the Gromov-Wasserstein distance. We derive for ß € [0; 1=2) distributional limits of this test statistic. To this end, we introduce a novel U-type process indexed in ß and show its weak convergence. Finally, the theory developed is investigated in Monte Carlo simulations and applied to structural protein comparisons.
Hongteng Xu (Duke University):
Gromov-Wasserstein Factorization for Graph Representation
Graph representation is significant for many real-world applications, e.g., network analysis, molecule clustering, and classification, etc. In this talk, I will introduce a new nonlinear factorization model, representing graphs that are with topological structures, and optionally, node attributes. This model is based on a pseudo-metric called Gromov-Wasserstein (GW) discrepancy, which compares graphs in a relational way. It achieves a novel and flexible factorization mechanism under the GW discrepancy, which estimates observed graphs as GW barycenters constructed by a set of atoms with different weights. The atoms and the weights associated with the observed graphs are learned by minimizing the GW discrepancy between the observed graphs and the barycenters of the atoms. I will show that this GW factorization model represents graphs with different sizes as vectorized permutation-invariant features. The learning algorithms of this model, its extensions, and potential applications will be discussed in-depth.
Lori Ziegelmeier (Macalester College):
Using Topology to Measure Dynamics of Time-Varying Systems
A time-varying collection of metric spaces as formed, for example, by a moving school of fish or flock of birds, can contain a vast amount of information. There is sometimes a need to simplify or summarize the dynamic behavior, and recently, topological tools have been applied to this purpose. One such method is a crocker plot, a 2-dimensional image that displays the (non-persistent but varying with scale) topological information at all times simultaneously. We use this method to perform exploratory data analysis and investigate parameter recovery in the collective motion model of D’Orsogna et al. (2006). Then, we use it to choose between unbiased correlated random walk models of Nilsen et al. (2013) that describe motion tracking experiments on pea aphids. Finally, we discuss an extension of the crocker plot that is persistent and equivalent to the information in a vineyard and hence, inherits the nice stability properties of vineyards. For some purposes, the information in a vineyard is more accessible when instead displayed in this new manner.