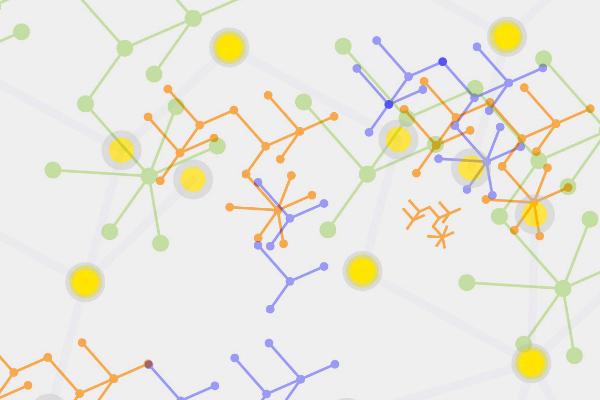
Topological and geometric ideas have already shown promises in producing novel perspectives and powerful algorithms for analyzing complex and diverse data. As the theory and foundations of topological data analysis continue to mature, we are presented with great opportunities to consolidate existing synergy as well as to establish new connections and collaborations among computational scientists, mathematicians, and statisticians, so as to form new perspectives and develop novel methodologies / algorithms for modern data analysis. This workshop, organized by the TGDA group at OSU, presents a timely platform to help achieve these goals.
Current PhD students and young researchers within 5 years of the PhD degree are qualified to apply for partial travel / accommodation support to attend the TGDA workshop.
Advisory Committee
Larry A. Wasserman, Statistics, CMU
Peter Bubenik, Mathematics, U. Florida
We very gratefully acknowledge funding and support from our NSF TRIPODS grant, our NSF RTG grant, The Ohio State Math Research Institute MRI and the Ohio State Mathematical Biosciences Institute MBI.